前言
说实话,之前我在笔记本上都一直都是只有一块N卡,所以没有过多关注过这个问题。然而昨天有个人问我,TensorRT怎么在多个GPU中指定模型推理GPU设备?我查了一下,发现官方有几个不同的解决方案,个人总结了一下,主要的做法有两种。
01配置环境变量支持
该方法的好处是不需要修改代码,通过配置环境变量就可以实现指定的GPU运行,缺点是缺乏灵活性,特别是想切换不同GPU实现模型推理的时候,这个方法就弊端就比较明显。
CUDA编程中支持的指定GPU设备的环境变量为:
CUDA_VISIBLE_DEVICES通过该系统的环境变量可以设置指定的单个GPU编号或者多个GPU编号合集,然后在程序测试与调试环境中使用。通过这种方式指定GPU编号执行模型推理,就无需修改代码,实现在单一指定的GPU上运行TensorRT推理程序。
02代码指定GPU设备执行
一台机器上可能有多个GPU设备,通过CUDA编程可以查询机器上所有的GPU设备,查询这些设备的属性以及决定使用哪个GPU设备作为当前设备。
cudaGetDeviceCount该函数可以查询到当前机器上GPU设备数目,然后遍历查询每个GPU设备的属性。官方教程给出的代码如下:
//查询设备数目 intdeviceCount; cudaGetDeviceCount(&deviceCount); //遍历设备编号信息 intdevice; for(device=0;device< deviceCount; ++device) { cudaDeviceProp deviceProp; cudaGetDeviceProperties(&deviceProp, device); printf("Device %d has compute capability %d.%d. ", device, deviceProp.major, deviceProp.minor); }根据查询的设备数目,GPU编号从0开始,默认情况下当前使用的设备就是编号为0的GPU设备,通过函数cudaSetDevice()可以修改运行时使用GPU设备,在初始化TensorRT之前,先通过cudaSetDevice()函数修改默认的当前设备,然后再初始化就可以把TensorRT的模型绑定到指定编号的GPU设备上推理。以我的笔记本上为例,设置当前的GPU设备,然后初始化TensorRT代码如下:
// 设置当前设备为GPU 0 cudaSetDevice(0);// 初始化TensorRT this->runtime=createInferRuntime(gLogger); assert(this->runtime!=nullptr); this->engine=runtime->deserializeCudaEngine(trtModelStream,size); assert(this->engine!=nullptr); this->context=engine->createExecutionContext(); assert(this->context!=nullptr); delete[]trtModelStream; // do more thing here// insert query input and output layers information //创建GPU显存输入/输出缓冲区 std::cout<< " input/outpu : " << engine->getNbBindings()<< std::endl; cudaMalloc(&buffers[input_index], this->input_h*this->input_w*3*sizeof(float)); cudaMalloc(&buffers[2],this->output_h*this->output_w*sizeof(float)); cudaMalloc(&buffers[1],32*25600*sizeof(float)); //创建临时缓存输出 prob.resize(output_h*output_w); mprob.resize(32*25600); //创建cuda流 cudaStreamCreate(&stream);在多个GPU设备上执行多个模型推理的初始化代码如下:
//初始化时间标记 cudaEvent_tstart,stop; cudaEventCreate(&start); cudaEventCreate(&stop); cudaEventRecord(start,0); //查询设备数目 intdeviceCount; cudaGetDeviceCount(&deviceCount); //遍历设备编号信息 intdevice; for(device=0;device< deviceCount; ++device) { cudaDeviceProp deviceProp; cudaGetDeviceProperties(&deviceProp, device); printf("Device %d has compute capability %d.%d. ", device, deviceProp.major, deviceProp.minor); } // Set GPU 0 as current cudaSetDevice(0); cudaStream_t s0; cudaStreamCreate(&s0); void* p0[1]; size_t size = 1024 * sizeof(float); cudaMalloc(p0[0], size); // initialization TensorRT here on GPU 0 // Set GPU 1 as current cudaSetDevice(1); cudaStream_t s1; cudaStreamCreate(&s1); // initialization TensorRT here on GPU 1 // 计算执行时间 cudaEventRecord(stop, 0); cudaEventSynchronize(stop); float elapsedTime; cudaEventElapsedTime(&elapsedTime, start, stop); printf("time to consume: %3.1f ms ", elapsedTime); // 销毁 cudaEventDestroy(start); cudaEventDestroy(stop);
关于延时加载
TensorRT8.6支持CUDA Lazy Loading(延时加载),开发者文档上说这种方式可以有效降低GPU显存与内存使用,加速初始化,节省模型初始化时间,可以通过环境变量配置实现延时加载支持,相关环境变量为:
CUDA_MODULE_LOADING=LAZY
-
gpu
+关注
关注
28文章
4733浏览量
128911 -
编程
+关注
关注
88文章
3614浏览量
93707 -
模型
+关注
关注
1文章
3233浏览量
48820 -
调试环境
+关注
关注
0文章
4浏览量
6005
原文标题:TensorRT | 在多个GPU中指定推理设备
文章出处:【微信号:CVSCHOOL,微信公众号:OpenCV学堂】欢迎添加关注!文章转载请注明出处。
发布评论请先 登录
相关推荐
如何在GPU上使用TensorRT部署深度学习应用程序
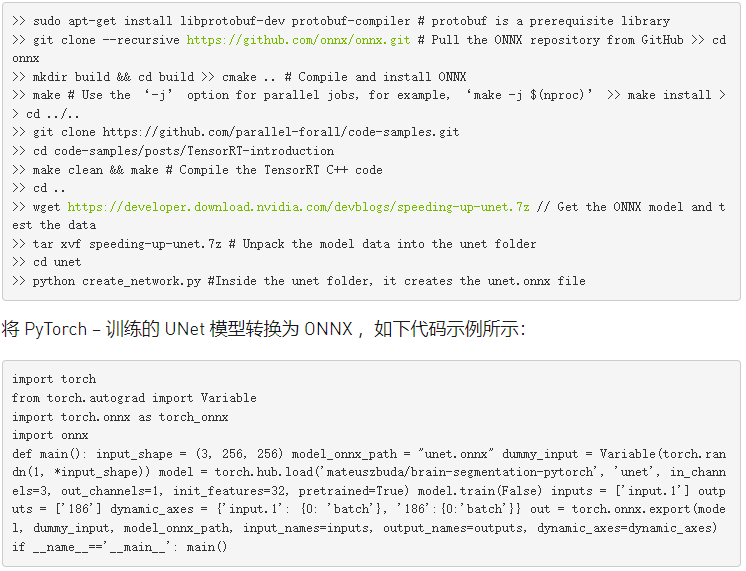
NVIDIA 在首个AI推理基准测试中大放异彩
TensorFlow指定CPU和GPU设备操作详解
充分利用Arm NN进行GPU推理
如何判断推理何时由GPU或NPU在iMX8MPlus上运行?
Keil中指定代码段与常量在Flash中地址方法
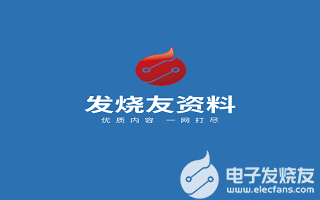
NVIDIA TensorRT 8.2将推理速度提高6倍
如何实现高效的部署医疗影像推理
NVIDIA TensorRT助力打造AI计算机视觉算法推理平台
NVIDIA T4 GPU和TensorRT加速微信搜索速度
NVIDIA TensorRT插件的全自动生成工具
学习资源 | NVIDIA TensorRT 全新教程上线
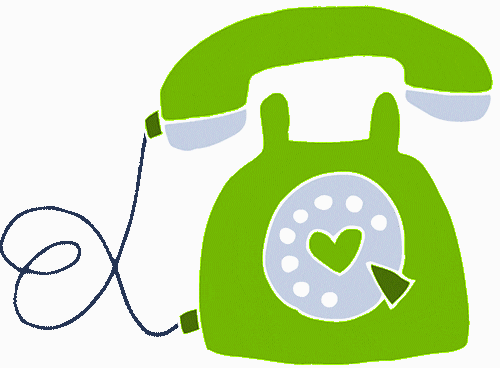
现已公开发布!欢迎使用 NVIDIA TensorRT-LLM 优化大语言模型推理
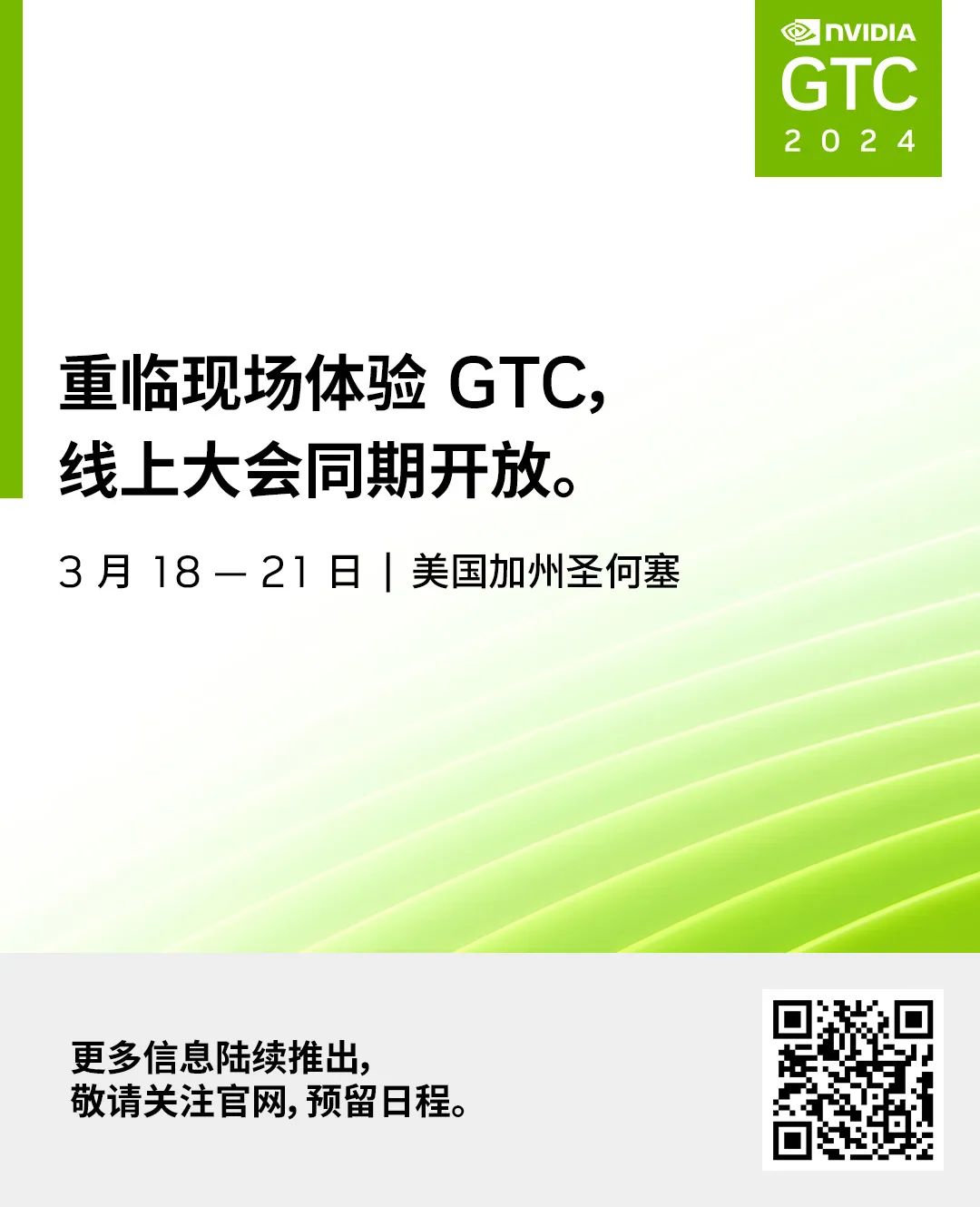
Torch TensorRT是一个优化PyTorch模型推理性能的工具
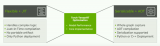
评论