近年来机器学习(ML)的快速发展使得基于第一性原理计算高效获得高精度分子动力学力场成为可能。目前人们已经发展了许多机器学习的力场interwetten与威廉的赔率体系 方法,由此实现了众多分子和固态系统的高精度计算,其精度接近密度泛函理论(DFT)等的量子力学方法,同时计算量显著降低。然而,当前大多数可用的ML力场只能给出能量、力和应力点的估算,而不是预测性分布,因而不能显示模型的不确定性。若没有模型不确定性估算,力场的拟合将费时费力,即需要从第一性原理计算数据库中手动或随机选择数千个参考结构来拟合。另外,在分子动力学模拟中,由于缺乏对模型不确定性的评估方法,将难以确定力场何时是可信赖的,从而导致结果的不可靠。
来自美国哈佛大学的Jonathan Vandermause和Boris Kozinsky共同领导的团队报道了一种基于机器学习的力场构建方法。该方法基于高斯过程回归的主动学习框架发展。其优势有二:其一,可以基于密度泛函理论(DFT)计算获得小数据集(~100个)来获得精确的力场;其二,通过误差估计可在偏离训练数据时自动进化。该优势使得该方法可以准确模拟和捕捉那些短暂且发生概率较低的原子事件。这些优势源于该模型使用了完全可解释的、低维的、非参数化力场。与经典的Stillinger-Weber力场类似,本研究使用的力场是基于多体原子间相互作用的,通常在2体和3体模型下即可具有足够的精度。基于此,可将描述符空间简化为一个低维空间,这一方面使得通过一组小的训练数据即可对全空间进行采样,另一方面也简化了学习任务,从而可以采用数据驱动的方式实现参数的自动调整。将该方法应用于含有单一或多种元素体系的分子动力学模拟,如铝晶体熔化、空位扩散和原子扩散、AgI中离子扩散等。结果表明,他们的方法能以低几个数量级的计算成本获得与DFT计算相当的精度,目前已完全在线开源发布。
On-the-fly active learning of interpretable Bayesian force fields for atomistic rare events
Jonathan Vandermause, Steven B. Torrisi, Simon Batzner, Yu Xie, Lixin Sun, Alexie M. Kolpak & Boris Kozinsky
Machine learned force fields typically require manual construction of training sets consisting of thousands of first principles calculations, which can result in low training efficiency and unpredictable errors when applied to structures not represented in the training set of the model. This severely limits the practical application of these models in systems with dynamics governed by important rare events, such as chemical reactions and diffusion.We present an adaptive Bayesian inference method for automating the training of interpretable, low-dimensional, and multi-element interatomic force fields using structures drawn on the fly from molecular dynamics simulations.Within an active learning framework, the internal uncertainty of a Gaussian process regression model is used to decide whether to accept the model prediction or to perform a first principles calculation to augment the training set of the model.The method is applied to a range of single- and multi-element systems and shown to achieve a favorable balance of accuracy and computational efficiency, while requiring a minimal amount of ab initio training data.We provide a fully open-source implementation of our method, as well as a procedure to map trained models to computationally efficient tabulated force fields.
责任编辑:pj
-
动力学
+关注
关注
0文章
105浏览量
16982 -
机器学习
+关注
关注
66文章
8423浏览量
132746
发布评论请先 登录
相关推荐
【Simcenter STAR-CCM+】通过快速准确的CFD仿真加速空气动力学创新
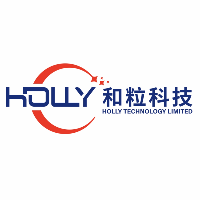
使用Phase Lab镍基动力学数据库计算多组分合金的成分分布曲线
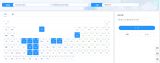
“本源悟空”超导量子计算机助力大规模流体动力学量子计算
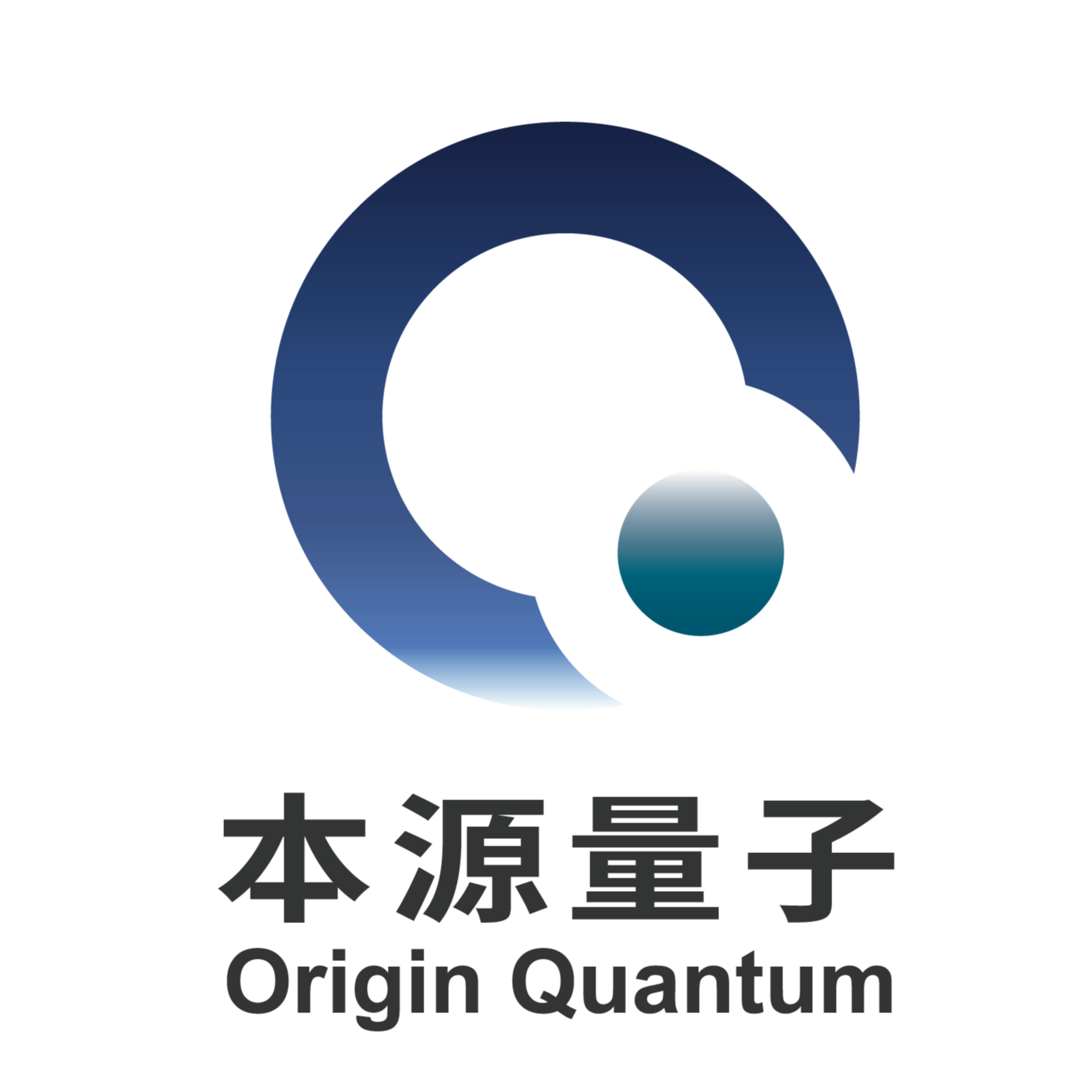
Simcenter STAR-CCM+车辆外部空气动力学特性——通过快速准确的CFD仿真加速空气动力学创新
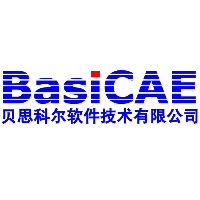
圆满收官| Aigtek参展第二届波动力学前沿与应用学术会议载誉归来!
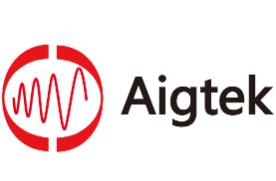
高精度电压源的作用、特点及应用介绍
邀请函| Aigtek安泰电子携经典产品,亮相第二届波动力学前沿与应用学术会议!
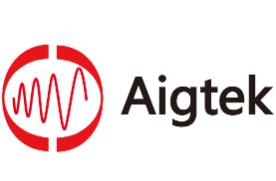
NVIDIA与百度飞桨携手革新汽车风阻预测:DNNFluid-Car模型的崛起
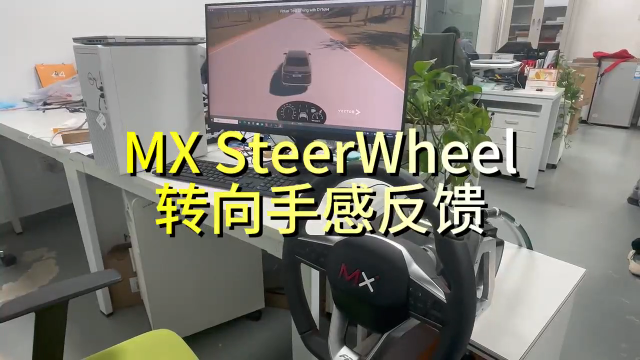
电感多少精度算高精度
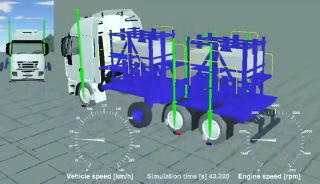
评论