1. 介绍
本文提出一种专门用于检测小目标的框架,框架结构如下图:
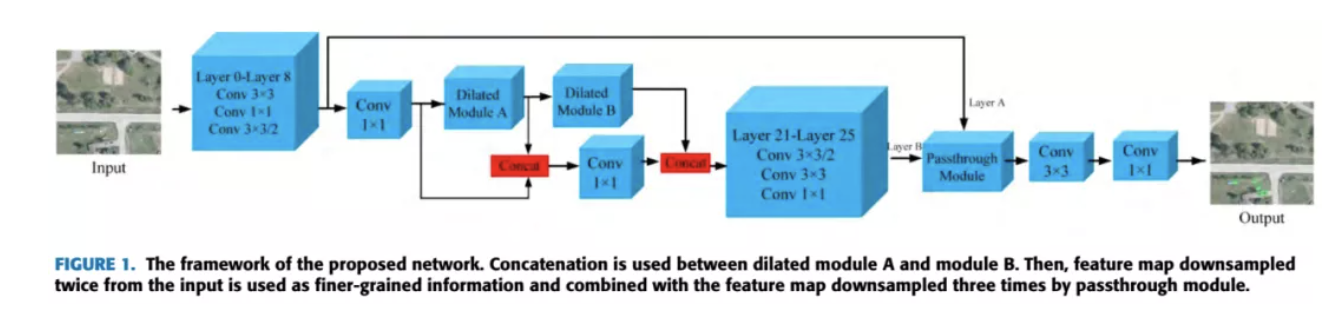
我们探索了可以提高小目标检测能力的3个方面:Dilated模块,特征融合以及passthrough模块。
Dilated Module :上下文信息对于检测小目标是很重要的,一种方法是重复的上采样来恢复丢失的信息,同时下采样来扩大感受野。但是,这个过程中信息丢失是难免的,受dilated卷积的启发,我们提出了Dilated Module,在不损失分辨率的情况下,提升感受野。
特征融合 :不同层的特征图包含不同的特征,浅层特征包含细节信息,深层特征包含语义信息,两者对于检测小目标都很重要,所以,对于不同的Dilated Module出来的特征图,我们进行拼接,全部用来检测小目标。
Passthrough Module :位置信息对于小目标也是至关重要的,我们提出了Passthrough Module从结合浅层特征图和深层特征图一起得到准确的位置信息。
本文的主要贡献:
(1)提出了Dilated Module来扩大感受野,提出了Passthrough Module来利用上下文信息和小目标的位置信息。考虑到小目标的特点,用特征融合来同时获取细节特征和语义信息,为了模型加速,使用了1x1卷积来降维。
(2)基于VEDAI数据集和DOTA 数据集,我们制作了一个small vehicle数据集,同时,分析了每个数据集的分布。
(3)我们在VEDAI数据集和DOTA 数据集上进行了训练和评估,结果表明,我们的网络非常的简单,快速和高效。
2. 方法
2.1 DILATED模块
使用Dilated卷积可以在不降低分辨率的情况下,指数的增加感受野,如图2所示:
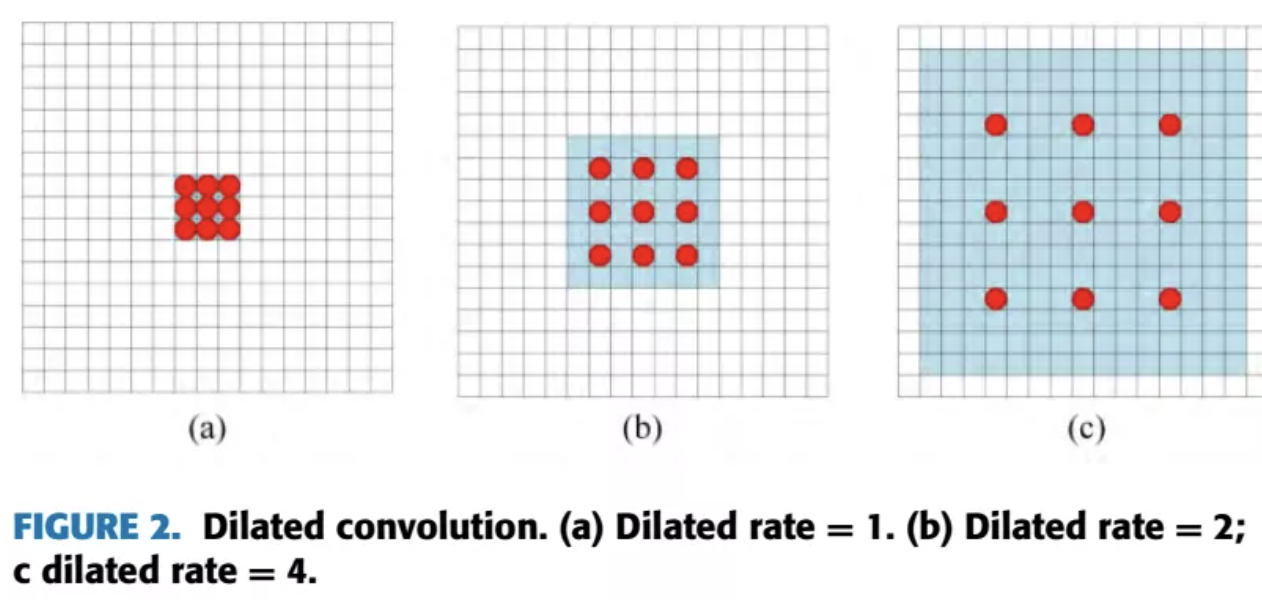
我们使用Dilated卷积作为基本单元,构建Dilated Module,为了重复使用之前层的特征,我们把之前层的特征通过拼接合并起来,然后使用1x1的卷积来降维,模块结构如下:
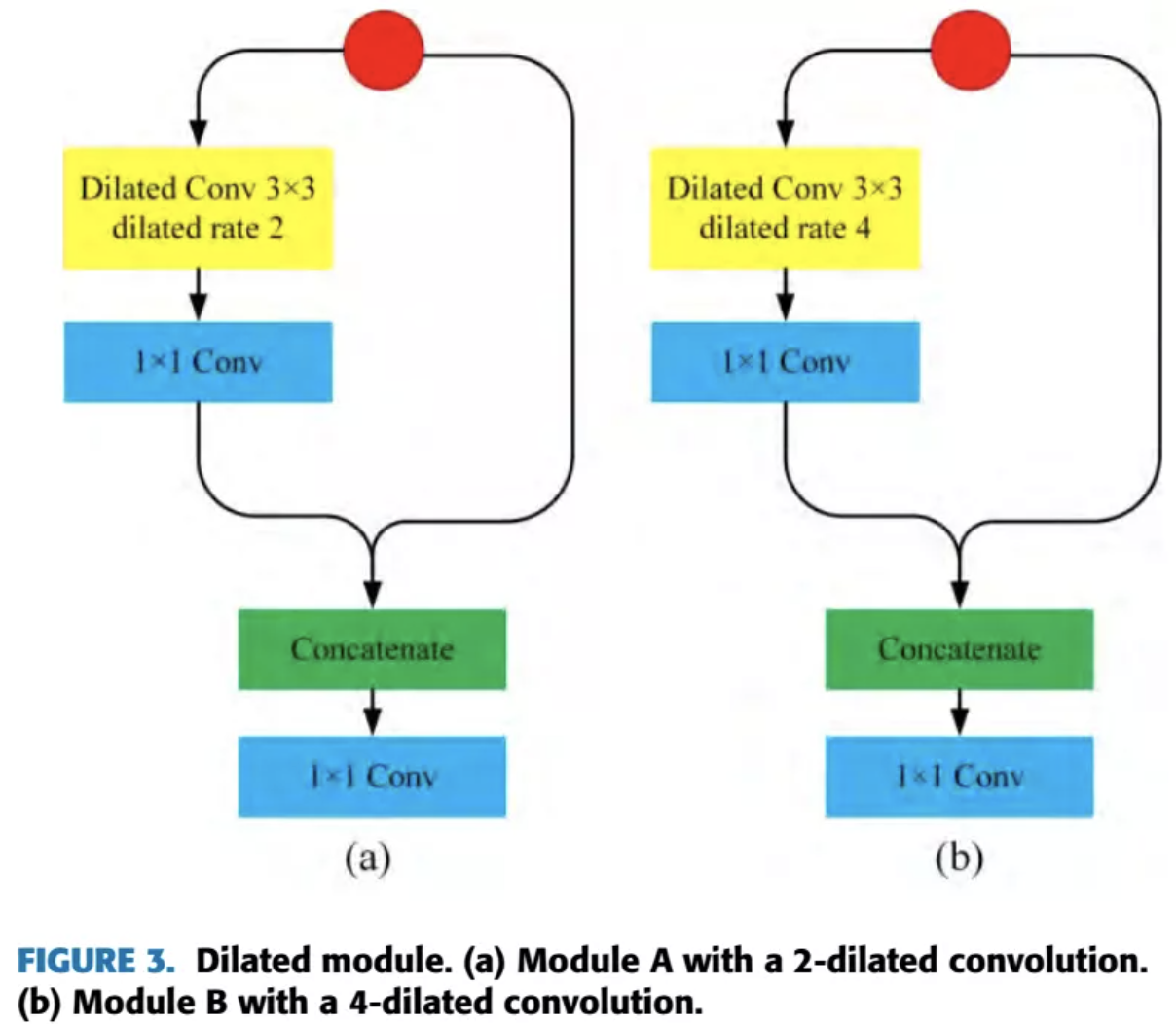
2.2 PASSTHROUGH模块
为了可以利用到之前的层的信息,我们使用了stride为2的passthrough层,这个passthrough层将特征图从2N×2N×C转换为N×N×4C,过程如图4,左边是passthrough层的输入,右边是passthrough层的输出:
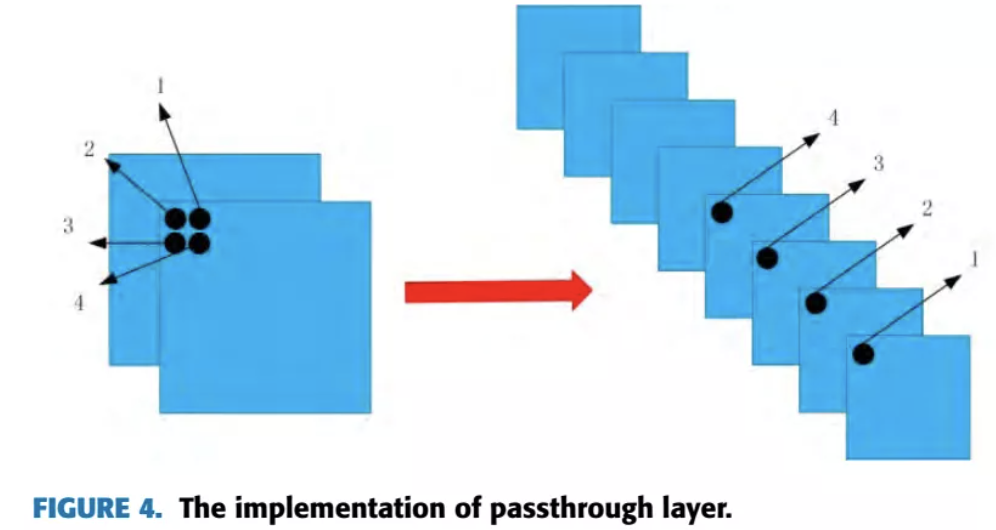
我们使用passthrough层作为基本单元,构建了passthrough module,具体结构见图5,LayerA表示之前的层,LayerB表示当前的更深一点的层:
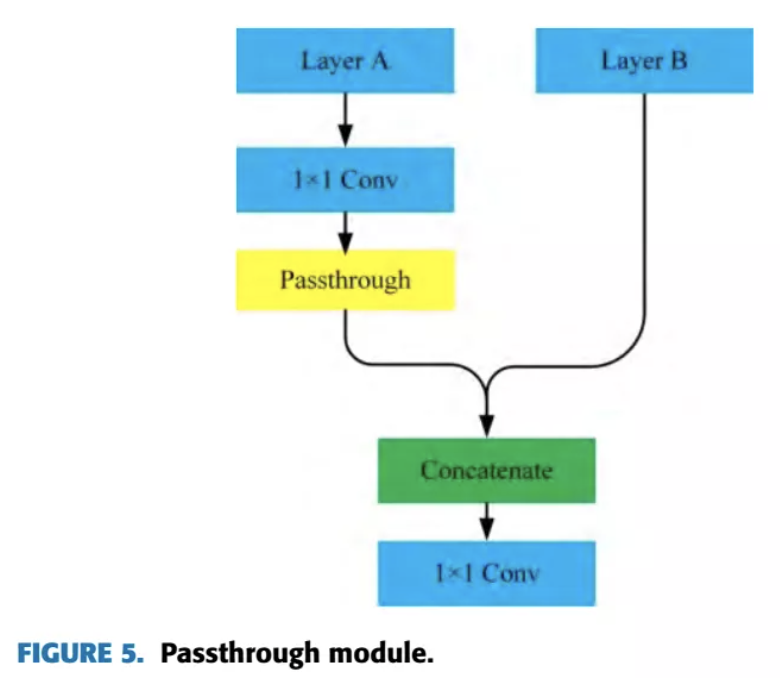
2.3 特征融合
本文使用拼接的方式来融合浅层特征和深层特征,框架里有2种特征融合的方法,一种是在不同的Dilated Module之间进行特征融合,此时,特征图的分辨率是相同的,直接拼接就可以。见图6。还有一种是类似passthrough module,中间有降采样的过程,特征图的分辨率已经改变了,如果要拼接的话,就需要进行通过passthrough层或者上采样层。这里选用了passthrough层来进行融合。
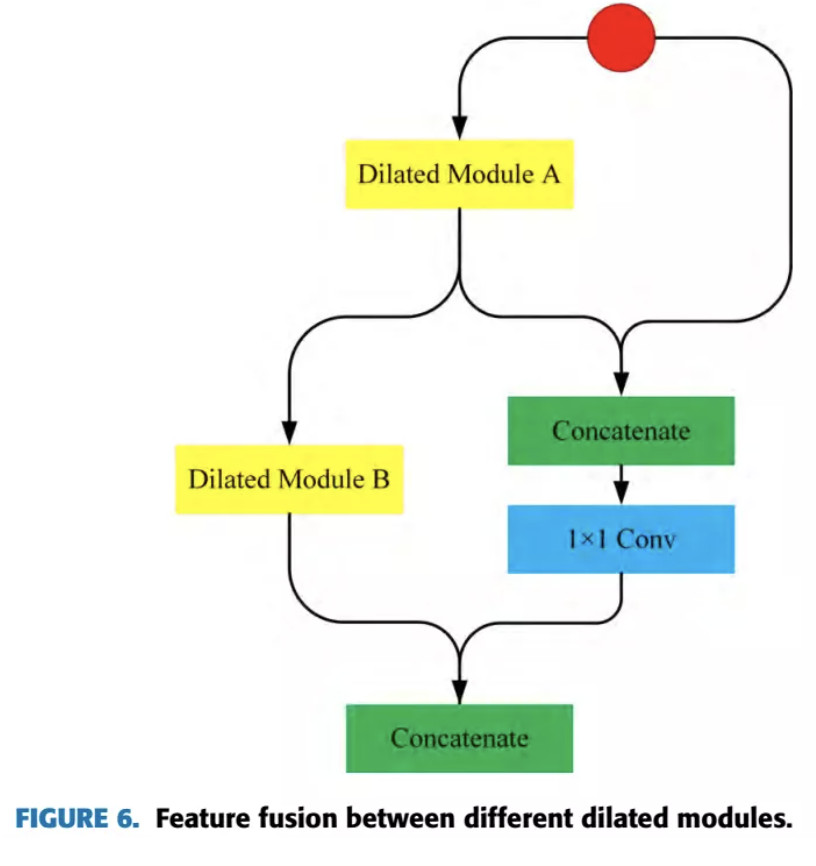
2.4 网络结构
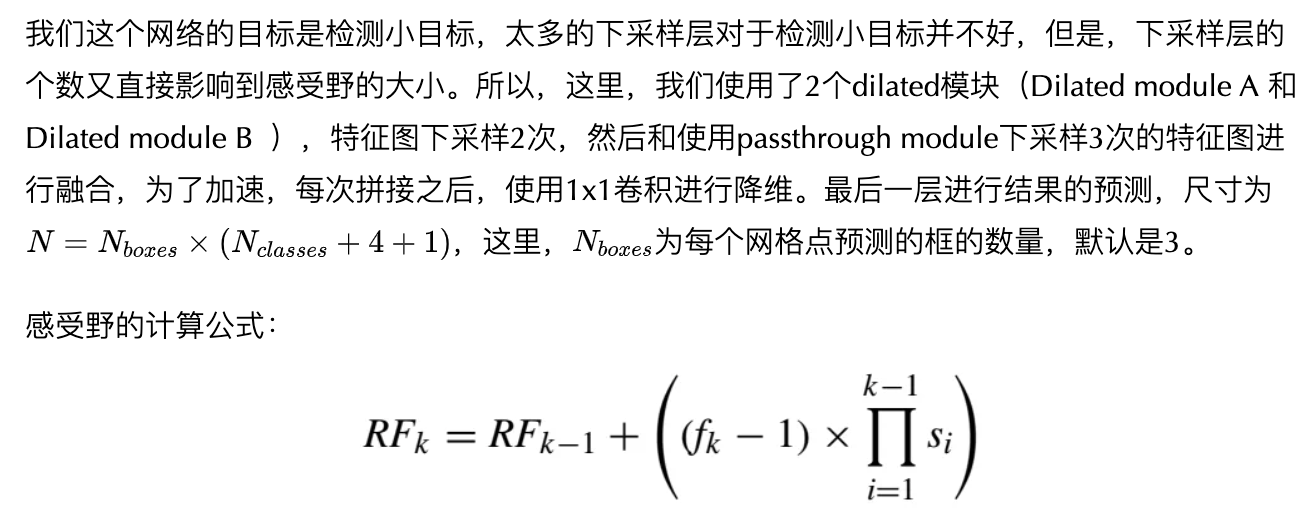
如表3所示,使用了2个Dilated卷积的网络,只下采样3次,感受野和下采样4次的网络一样。
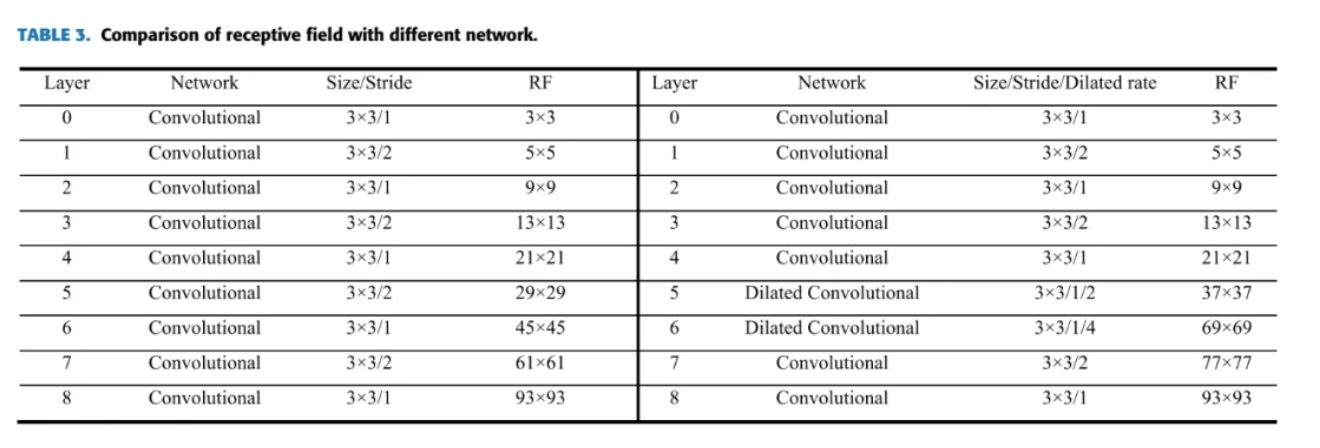
3 实验
我们分析了VEDAI数据集和DOTA 数据集的数据分布:
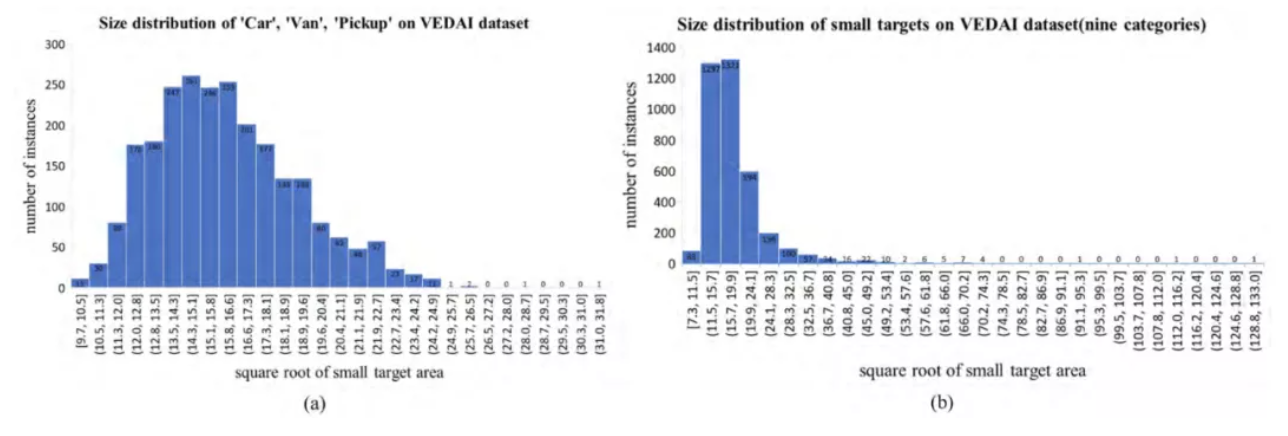
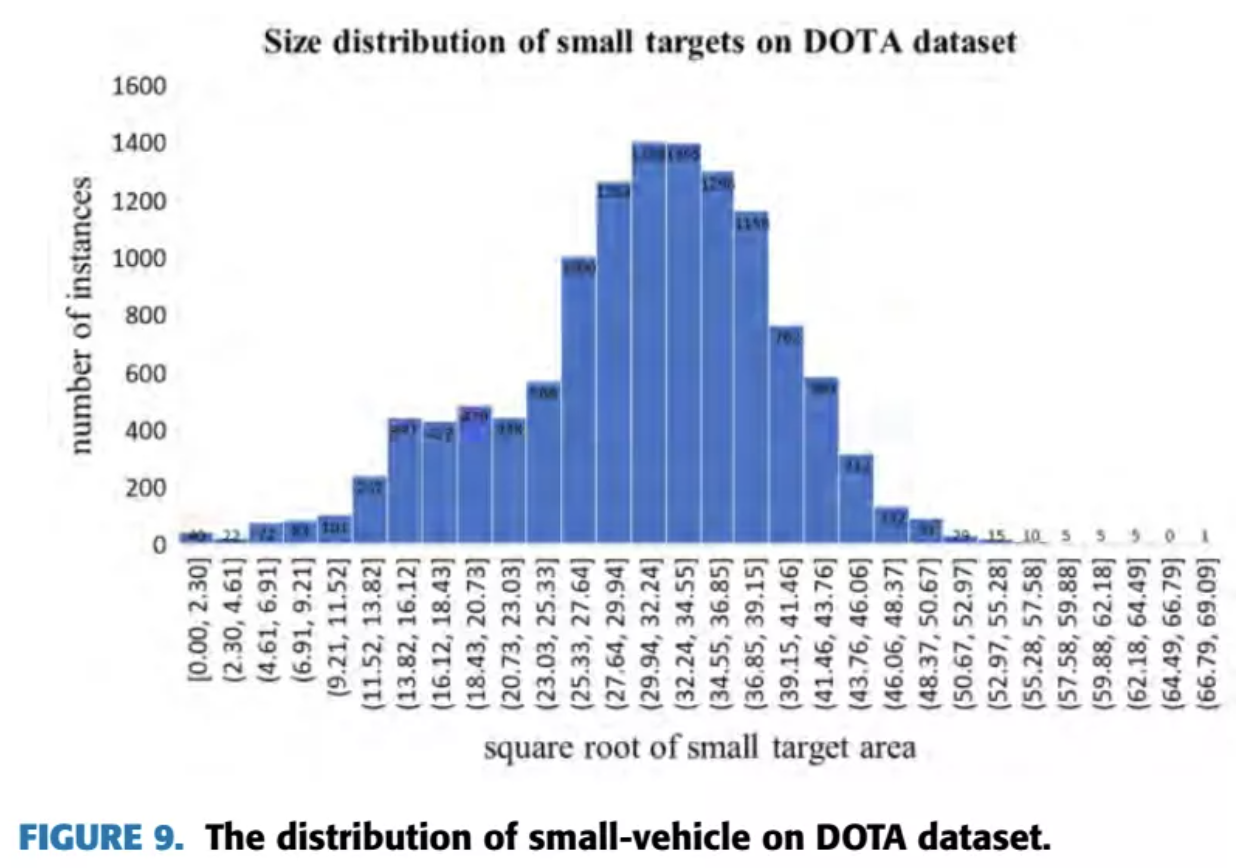
在VEDAI数据集上,设计了2种实验,实验1是将‘car’, ‘pick-up’和 ‘van’ 合并成了1个类别,实验2是使用了原来的9个类别,结果如下,结果明显好于YOLOv2和Tiny YOLOv3,但是低于YOLOv3:
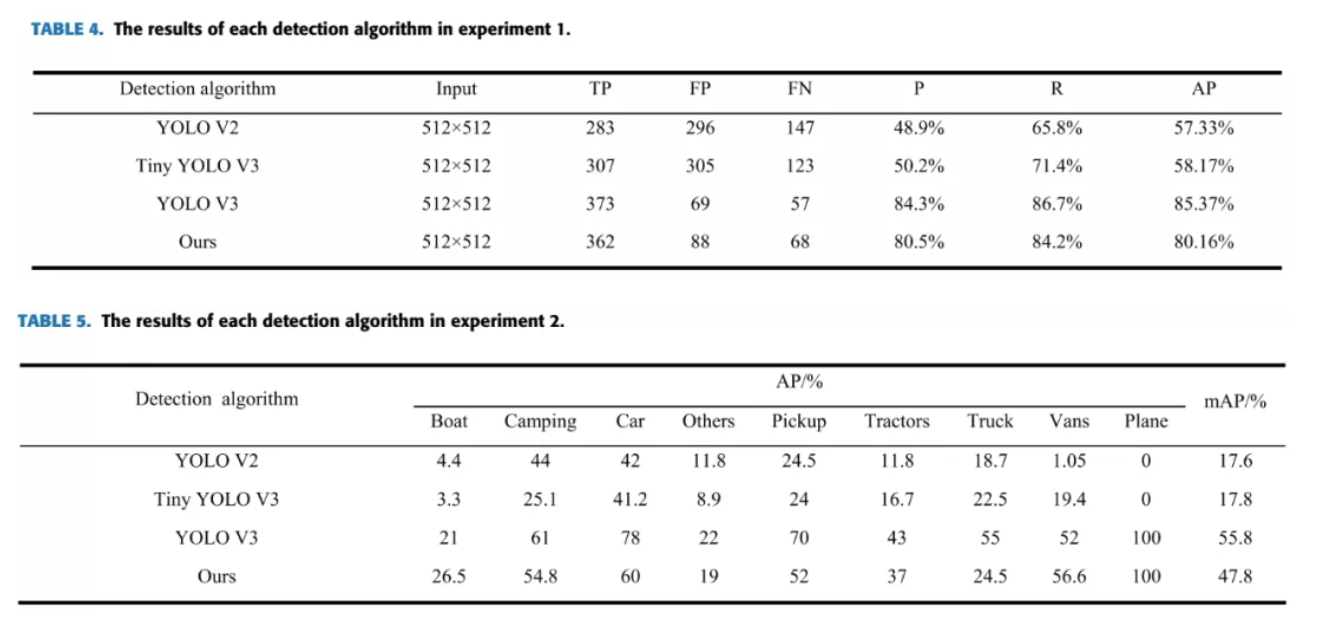
在DOTA数据集上的比较:
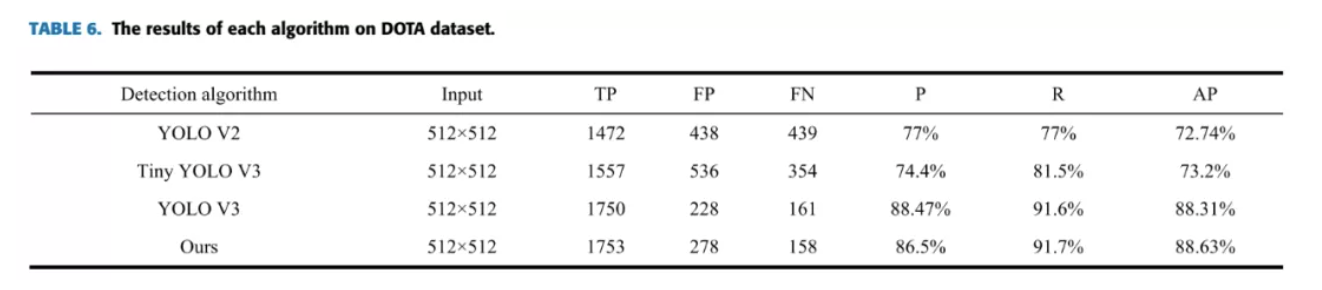
不同网络的速度的比较:
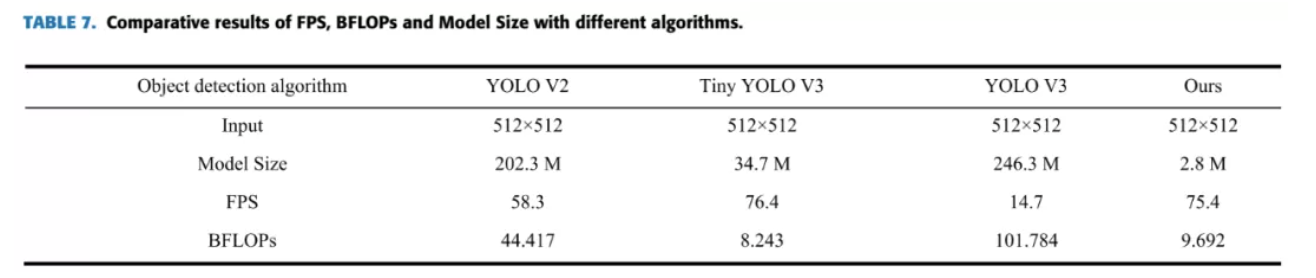
可以看到,模型速度和Tiny YOLOv3相当,准确率大大超过Tiny YOLOv3,仅仅比YOLOv3略低。
原作者:ronghuaiyang